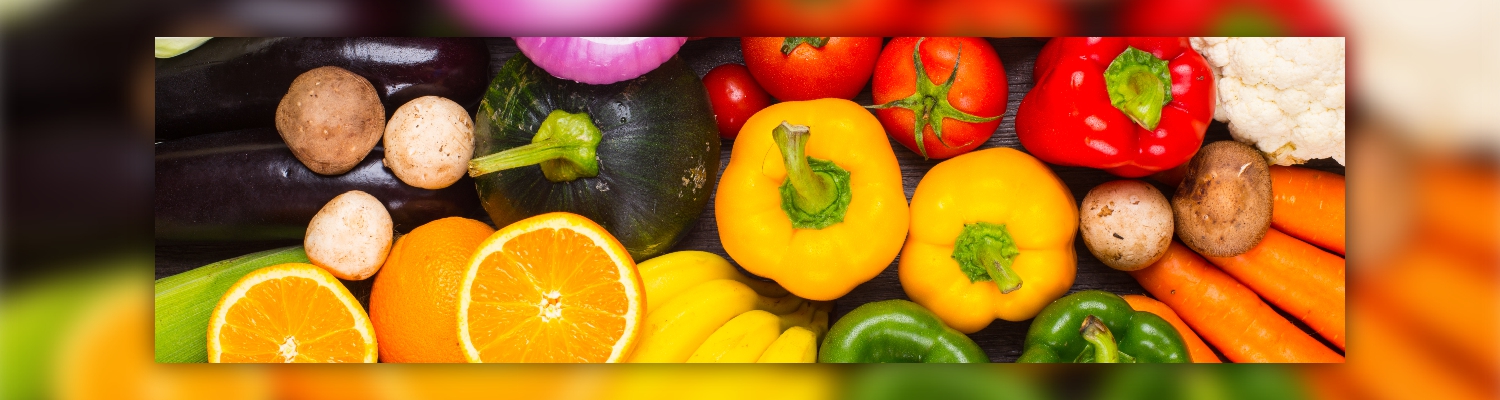
ISSN: 2638-6070
Ashenafi Getachew, Kefelegn Kebede*, Mengistu Urge and Yesihak Yusuf Mumed
Received:June 19, 2023; Published:July 11, 2023
*Corresponding author:Kefelegn Kebede, School of Animal and Range Sciences, Haramaya University, Haramaya, Ethiopia
DOI: 10.32474/SJFN.2023.04.000200
Two exotic chicken breeds, namely Sasso, and White Leghorn were used in this study with the objective to unfold the interdependence among egg quality traits, and to predict egg weight from their orthogonal egg quality traits using principal component regression. Traits measured included egg weight (EW), egg length (EL), egg width (EWi), albumen height (AH), yolk height (YH), albumen weight (AW), yolk weight (YW), yolk colour (YC), and eggshell weight (SW). Traits were measured on eggs obtained from 633 eggs of Sasso, and 1023 eggs of White Leghorn. Pearson correlations between egg weight and egg quality traits were positive and highly related (r = 0.614-0.937 in Sasso, and 0.496-0.943 in White Leghorn breeds respectively). In factor solution of the PCA with varimax rotation of the transformation matrix, three principal components (PCs) were extracted (PC1, PC2 and PC3) explaining 69.2% (Sasso) and 68.5% (White Leghorn) of the total variation in the original variables. In principal component regression models, 73% (Sasso) and 69% (White Leghorn) of the variability in egg weight is accounted by the model used. It can be concluded that the use of principal components’ scores from chickens’ egg quality traits was more appropriate than the use of original traits in egg weight estimation.
Keywords:Chicken Breeds; Egg Quality Traits; Principal Component; Regression
Chicken eggs are an excellent source of protein, with a low energy content, and are well-balanced in their nutrient content [1]. Egg quality traits are very important characteristics that influence the quality, grading and weight of newly hatched chicks; their hatching performance; and consequently, the economic outcome of the enterprise [2-4]. The limited genetic potential of the native chicken breeds, is the main cause of the poor output performances [5].The per capita consumption of chicken eggs (kg/person/year) is 0.66 [6]. The need for superior chicken that combines higher production and better adaptation has grown recently. The African Chicken Genetic Gain project is now working in three African nations-Ethiopia, Nigeria, and Tanzania- to test imported exotic chicken breeds. However, performance assessment is crucial to promoting the production and dissemination of these breeds on a big scale. So far, some research studies on egg quality traits of exotic chicken breeds have been done in Ethiopia [7,8]. However, the data used were of a small sample size and the evaluation was made using uni(bi)variate analysis (analysis of variance, and correlations). The mechanisms underlying egg quality traits are too complex to be explained using uni(bi)ivariate analysis because, on the one hand, each trait is examined separately, resulting in significant overlap of results, and, on the other hand, egg quality traits are biologically related due to pleiotropy or linkage [9-12]. Consequently, there is a lack of information on the use of principal component regression (PCR) to estimate egg weight from egg characteristics. Therefore, the main goal of this study was to better understand the complex interactions between the features that determine egg quality and to examine these relationships using orthogonal conformation traits that were obtained from PC component scores. The information gathered will help with breeding programme development, management, and conservation.
Study Area Location
The study was undertaken at the poultry research farm of Haramaya University, which is located at an altitude of 1980 meters above sea level, 4°26’N latitudes and 4203’E longitude. The mean annual maximum and minimum temperatures are 23.4°C and 8.25°C, respectively and the area has an average annual rainfall of 780 mm.
Experimental Animals and Their Management
Two exotic chicken breeds (i.e., Sasso and White Leghorn) were used for this study that were reared under similar housing and feeding management conditions. A total of 1656 eggs (633 from Sasso, and 1023 from White Leghorn) were used. The chickens providing the eggs were of the same age (hatched on the same day) and egg collection occurred when the hens were 21–72 weeks of age and data on egg quality parameters were recorded on the same day of collection. The chickens were kept in brooder houses with incandescent heating lamps for the first eight weeks. Afterwards, they were reared under the grower house, during the growing period and in the layer house of deep litter system during the laying period. They were offered clean drinking water ad libitum and the recommended level of feed (i.e., a standard ration of 20% crude protein (CP) and 2800 Kcal/kg metabolizable energy(ME) during the first eight weeks, 16% CP and 2800 Kcal/kg ME during the growing period (9 to 20 weeks), and 16.50% CP and 1750 Kcal/ kg ME during laying stage) according to their requirement. All the necessary vaccines were administered for the chicken at the appropriate ages.
Traits Measured
External (egg weight (EW), shell weight (SW), egg length (EL),
and egg width (EWi)) and internal (albumen height (AH), albumen
weight (AW), yolk height (YH), yolk weight (YW), yolk colour (YC))
egg quality traits were measured.
a) Albumen height (AH), was measured between the yolk and
outer edge of the thick albumin in millimetre (mm) with the
help of Spherometer.
b) Albumen weight (AW), was measured in gram (g) with the
help of electronic balance with 0.1g accuracy.
c) Egg length (EL), measured in centimetre (cm) with the help of
Vernier calliper.
d) Egg weight (EW), measured in gram (g) with the help of electronic
balance with 0.1g accuracy.
e) Egg width (EWi), measured in centimetre (cm) with the help
of Vernier calliper.
f) Shell weight (SW), measured in centimetre (cm) with the help
of Vernier calliper.
g) Yolk colour (YC), was examined by using yolk colour fan
(ROCHE, Yolk Colour Fan, Switzerland) and the most appropriate
colour number was given accordingly.
h) Yolk height (YH), was measured in millimetre (mm) with the
help of Spherometer.
i) Yolk weight (YW) was measured in gram (g) with the help of
electronic balance with 0.1g accuracy.
SAS-program version 9.4 [14] was used for all statistical analyses in this study.
Univariate Analysis: Egg quality traits(EW, AH, AW, YH, YW, SW, YC, EL, and EWi) were subjected to independent t-Test analysis of variance using SAS to determine the effect of breed.
The linear model employed was:
where:
Yij = Observed value of the egg quality trait
μ= Overall mean
Bi= Fixed effect of the ith breed (i = 2:1=Sasso, and 2=White
Leghorn)
εij= Random residual error term
Multivariate Analysis: Pearson correlation coefficients among the egg quality traits were calculated for each breed and the correlation matrix was generated as the primary data required for PCA. PCR is an appropriate multivariate technique to reduce the dimension of a data set consisting of a large number of interrelated variables, while retaining as much as possible the variation present in the data set [15]. This is achieved by transforming a set of original variables to a new set of variables, the principal components which are ordered so that the first few retain most of the variation present in all of the original variables [16]. PCA is a method for transforming the variables in a multivariate data set x1, x2, …, xp, into new variables, y1, y2, …, yp which are uncorrelated with each other and account for decreasing proportions of the total variance of the original variables as defined below:
The aim of the varimax rotation is to maximize the sum of variances of aij2 quadratic weight.
A multiple regression procedure was used to obtain models for predicting egg weight from egg quality traits (a) and PCA regression from established principal components (b).
where; EW is the egg weight, b0 is the regression intercept, bi is the ith partial regression coefficient of the ith egg quality trait, Xi or the ith principal component. Anti-image correlations and Barlett’s Test of Sphericity were computed to test the validity of the factor analysis of the data sets. The appropriateness of the factor analysis was further tested using communalities. Cumulative proportion variance was employed in determining the number of principal components to extract. The overall reliability of the factor solution was tested using Chronbach’s Alpha. The means, correlation, factor, and regression procedures of SAS 9.4 statistical package was used for the analyses.
Univariate Analysis: Least-square means (LSM) along with their standard error (SE) of the egg quality traits are presented in Table 1. All the traits showed a wide range of variability between the breeds. The Sasso breed had significantly (p < 0.05) higher mean values than the White Leghorn breed in all the investigated traits. The significant variation in the observed traits could be attributed to the genetic make-up of the breeds. The result is in line with [7] and [8] who reported the effect of breed on egg quality traits.
Multivariate Analysis: Table 2 shows the correlation coefficients of egg quality traits of the exotic chicken breeds. The correlation coefficients ranged from 0.00 to 0.75, and -0.01 to 0.78 in Sasso, and White Leghorn breeds respectively.
a,b when different superscripts are indicated in the same row for a given trait, it means that there is a significant (P< 0.05) effect of breed. EW = egg weight, AH = albumen height, AW = albumen weight, YH = yolk height, YW = yolk weight, SW = shell weight, YC = yolk colour, EL = egg length, and EWi = egg width.
Table 2: Pearson correlations among egg quality traits of exotic chicken breeds of Sasso (above diagonal), and White Leghorn (below diagonal).
* = significant at alpha values of 0.05; ns =not significant; EW = egg weight, AH = albumen height, AW = albumen weight, YH = yolk height, YW = yolk weight, SW = shell weight, EL = egg length, and EWi = egg width.
PC Factor Analysis: Anti-image correlations computed (not given here) showed that partial correlations were low, indicating that true factors existed in the data. This result is consistent with that of the Kaiser-Meyer-Olkin (KMO) measure of sampling adequacy studied from the diagonal of partial correlation, revealing the proportion of the variance in the traits caused by the underlying factor [17]. The KMO measure of sampling adequacy was found to be sufficiently high with a value of 0.78. [18] reported that a KMO measure of 0.60 and above is considered adequate. Bartlett’s sphericity test for testing the null hypothesis that the correlation matrix is an identity matrix was used to verify the applicability of PCA. The value of Bartlett’s sphericity test was significant (p-value = 0.001), implying that the PCA applies to the data set.
Eigenvalues, Percentage of Total Variance with Rotated Component Matrix and Communalities
The eigenvalue of the total variance, the rotated component matrix and communalities of the traits investigated are presented in Table 3. The table shows how much of the total variance of the observed traits was explained by each of the PCs after varimax rotation of the component matrix. For the Sasso breed, three PCs were identified with eigenvalues of 1.87 (PC1), 1.20 PC2, and 1.09 (PC3). PC1 explained 312%, PC2 explained 20%, and PC3 explained 18.1% of the total variance respectively. Similarly, for the White Leghorn breed, three PCs were identified with eigenvalues of 1.80 (PC1), 1.42 PC2, and 0.89 (PC3). PC1 explained 30%, PC2 explained23.7%, and PC3 explained 14.8% of the total variance respectively. Communalities are the proportion of variance that each trait has in common with other traits. Thus, if communality of a trait is high, it means that the extracted factors explained a big proportion of the variance of the trait. For the Sasso breed, the communality values ranged from 0.15 (YC) to 1.00 (AH); while for the Whit Leghorn breed the values ranged from 0.16 (YH) to 1.00 (AH). PC loadings presented in Table 3 are the correlation coefficient between the first three PC scores and the original traits. They measure the importance of each egg quality trait in accounting for the variability in the PC.
Table 3: Eigenvalues and share of total variance along with factor loadings after rotation and communalities of the egg quality traits of the chicken breeds.
EW = egg weight, AH = albumen height, AW = albumen weight, YH = yolk height, YW = yolk weight, SW = shell weight, YC = yolk colour, EL = egg length, and EWi = egg width.
That is, the larger the loadings in absolute terms, the more influential the variables are in forming the new PC and vice versa. For the Sasso breed, the first factor (PC1) loaded heavily on AW (0.63), and YW (0.60) and SW (0.66), the second factor (PC2) loaded heavily on AH (1.00), while the third factor (PC3) loaded heavily on YC (0.38). For the White Leghorn breed, the first factor (PC1) loaded heavily on AW (0.84), YW (0.73) and SW (0.32), the second factor (PC2) loaded heavily on AH (0.98), while the third factor (PC3) loaded heavily on YH (0.39), SW (0.39) and YC (0.46). A scree-parallel analysis plot of eigenvalues against their PCs is shown in (Figure 1a and 1b) below. This enhanced plot shows the rate of change in the magnitude of the eigenvalues for an increasing number of PCs. The rate of decline levels off at a given point in the scree plot that indicates the optimum number of PC to be extracted. Also, the intersection point between the scree plot & the parallel analysis plot reveals that the first three eigenvalues that account for could be retained as significant PC for both breeds (Figures 1a & 1b).
MLR Models Used for Body Weight Prediction of Chickens
The interdependent original egg quality traits and their independent PC factor scores were used to predict egg weight. Tables 4a & 4b present the regression coefficient, their standard errors, t-value, p-values, variance inflation factor (VIF) values, and R2 obtained from MLR analysis. For the Sasso breed, the regression of egg weight on AW, YW and SW were significant, while it was not significant for AH, YH and YC. For the White Leghorn breed, the regression of egg weight on AH, AW, YW and SW were significant, while it was not significant for YH and YC. The present findings are consistent with the submissions of [19] in chickens and [20] in ducks. To increase egg yields from chicken production, the genetic improvement of egg weight is necessary and this requires adequate knowledge of correlated traits that can be considered when selection is to be applied. However, the use of inter-dependent predictors should be treated with caution, since multi-collinearity is associated with unstable estimates of regression coefficients [21-23] rendering the estimation of unique effects of these predictors impossible.
This was not the case in the present study, where VIF values greater than 10 were not found. [24] stated that VIF values above 10 indicate severe collinearity which leads to unstable estimation of the associated least square regression coefficient. To overcome this limitation, the PC factor scores are used as predictors for the prediction of egg weights [25, 23]. These PCs are orthogonal to each other and are more reliable in weight estimation. In the present study, the use of PC1, PC2 and PC3 as multiple predictors explained 73% and 69% of the total variability in egg weight of Sasso and White Leghorn breeds respectively. For the Sasso breed the two factors selected (PC1 and PC2) were found to have a significant (p < 0.0001) positive linear relationship with egg weight; while for White Leghorn breed all three PCs were significant (Table 4a and 4b). In other words, egg weight is expected to increase as the values of factor 1 and 2 scores in Sasso increase; while for White Leghorn, egg weight is expected to increase as the values of factor 1, 2 and 3. Similarly, [26] derived regression equations for estimating the egg weight of chickens using independent factor scores. In another related study, [27] obtained a regression model for body weight prediction using principal component scores of musk ducks; while [28] used factor scores derived from egg quality traits to predict the egg weight of chicken.
Table 4a: MLR of egg weight on original egg quality traits of Sasso breed and their PC factor scores.
Table 4b: MLR of egg weight on original egg quality traits of White Leghorn breed and their PC factor scores.
In this study, PC factor analyses were explored in identifying patterns and determine the inter-dependence in seven egg quality traits of exotic chicken breeds to eliminate redundancy and predict egg weight. According to the results of this study, it could be concluded that using inter-dependent egg quality traits as predictors in MLR analysis resulted in a multi-collinearity problem. On the other hand, when independent orthogonal indices (PC factor scores) were used as predictors, problem of multi-collinearity was removed. The results of this study imply that egg weight can be estimated more accurately from principal component (PC) factor scores and the results obtained could be used by poultry producers and researchers for selecting, managing, and estimating market values of chickens. This is because egg weight is the pivotal determinant for thriving animal production. However, further research is needed to investigate the relationship between egg weight with egg quality traits in the same and other ecotypes of chickens in the different region of the country with additional traits of egg quality parameters.
Haramaya University is acknowledged for supporting the research financially. Moreover, the authors are grateful to the district and Kebele office authorities and data collectors and respondents involved in this study.
Bio chemistry
University of Texas Medical Branch, USADepartment of Criminal Justice
Liberty University, USADepartment of Psychiatry
University of Kentucky, USADepartment of Medicine
Gally International Biomedical Research & Consulting LLC, USADepartment of Urbanisation and Agricultural
Montreal university, USAOral & Maxillofacial Pathology
New York University, USAGastroenterology and Hepatology
University of Alabama, UKDepartment of Medicine
Universities of Bradford, UKOncology
Circulogene Theranostics, EnglandRadiation Chemistry
National University of Mexico, USAAnalytical Chemistry
Wentworth Institute of Technology, USAMinimally Invasive Surgery
Mercer University school of Medicine, USAPediatric Dentistry
University of Athens , GreeceThe annual scholar awards from Lupine Publishers honor a selected number Read More...