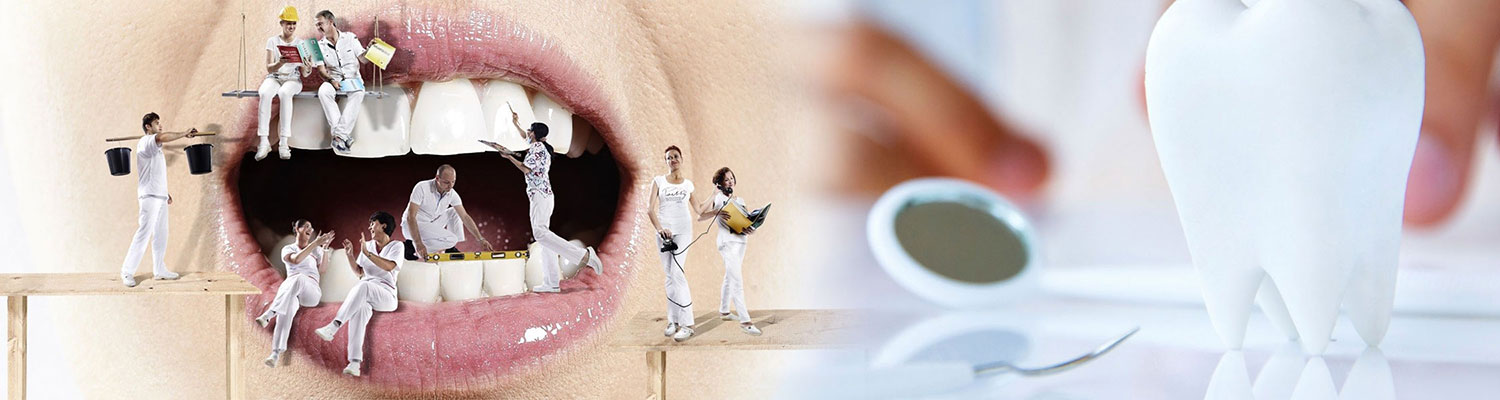
ISSN: 2637-4692
Dipankar Bandyopadhyay1*, Patrick Hilden2, Debdeep Pati3, Jyotika Fernandes4, Stephanie L Russell5, Jeffrey L Fellows6 and Radha Nagarajan7
Received: November 1, 2021 Published: November 11, 2021
Corresponding author: Dipankar Bandyopadhyay, Department of Biostatistics, Virginia Commonwealth University, Virginia
DOI: 10.32474/MADOHC.2021.05.000204
Count data abounds in various epidemiological, biological, or clinical settings, and are routinely analyzed using a Poisson or Negative Binomial distribution. The ability to accurately analyze and interpret such data remains an important area of research. In dental caries epidemiology, the DMFT/S index, which is the total number of (D)decayed, (M)issuing and (F)filled (T)teeth, or (S) surfaces, stands out as the single most important marker to quantify overall caries experience. However, while analyzing toothlevel caries responses, we often observe an excess of lower and upper bounded count values, such as 0, and accounting for these excess values remains a key component in selecting the most appropriate biostatistical modeling strategy. The aim of this paper is to compare the effectiveness of Binomial (B), Beta-Binomial (BB), and 2-part Hurdle- Binomial (HB) mixed effects models in analyzing tooth-level dental caries data derived from a clinical study of Type-2 diabetic Gullah speaking Africans residing in the coastal South Carolina (SC) sea-islands. All analyses were conducted using the SAS NLMIXED procedure which provides for flexible model specification allowing for direct fitting of more intricate models such as the BB, 2-part HB model, and other such models for which standard SAS functions do not exist, but for which a likelihood function is known. Our analysis found the BB mixed-effects model to be the most effective. Analysis reveal that for every 1-year increase in age, there is a 4.9% increase (p<0.05) in the mean probability of obtaining an additional DMFS. Furthermore, there is a decrease of 84.6%, 96.9%, and 94.2% (p < 0.05 for all three), respectively for premolars, canines and incisors, in the expected probability of an additional DMFS, compared to the molars. Results from this analysis should provide interesting insights into assessing complex covariate-response relationships in dental caries and should provide some guideline into effective statistical model choices.
Keywords: Caries; Count Data; Tooth-Level, Gullah, SAS Proc NLMIXED
Discrete count data abounds in a variety of scientific fields such as epidemiology, medicine, biology, and in a number of clinical trial settings [1,2]. For example, in the field of dental caries, we work with the popular DMFT/DMFS index [3] that counts the total number of decayed (D), missing (M) and filled (F) tooth(T)/surfaces(S) for the whole mouth. A common feature of these data is the presence of ‘overdispersion’ when fitting a Poisson (P) distribution, in the sense that the sample variance is larger than the sample mean, and hence the well-known “unit variance to mean ratio” is violated. Overdispersion is caused due to several factors, such as unobserved heterogeneity, missing covariates, or correlation among repeated or longitudinal measures. These count responses can also be characterized by excessive observations at one end of the ordering, typically, zeroes [4]. In caries DMFS, these zeros represent the cases where one does not observe any disease. Modeling strategies that account for overdispersion and excess zeroes continue to remain an important area of statistical research, particularly in oral epidemiology. Often, one may choose to use a Negative Binomial (NB) regression [5] to model full mouth (Poisson distributed) DMFS to tackle overdispersion. In situations of excess zeros, the zeroinflated (ZI) model as proposed by Lambert [6] is widely used in oral health studies [7]. In the ZI framework, the probability of being an excess zero is modeled through a mixture distribution allowing greater weight to be placed on the probability of observing a zero count [8]. A very nice review on applications of the ZI model to fullmouth DMFT/DMFS data, and some recommendations appear in Preisser et al. [9]. When modeling ZI, it is of utmost importance to consider the latent process for which the value of zero was attained. As an example, when considering the DMFT/DMFS for any population, there might be some tooth/surfaces which might remain potentially ‘disease-free’, while others are ‘disease free’ for the present and might have developed caries earlier but the lesions are no- longer active or are prone to develop carious lesions in the future. Those zeros arising from tooth/surfaces that are never truly at any risk are known as ‘structural zeros’, while zeros arising from tooth/surfaces potentially at risk contributes to ‘sampling zeros’ [4]. Within many data analysis problems, one can assume a latent process (which needs to be estimated) that divides the entire set of zeros into the structural and sampling components. The ZI modeling is often more advantageous if a dataset contains both these types of zeros, since the probabilities of both these types of zeros are modeled separately [8]. In cases with only an excess of sampling zeros, Hurdle(H) models proposed by Mullahy [10] are more appropriate. In contrast to the mixture setup in a ZI model, the H model is essentially a 2-part model, with the first part modeling a binary response, and the second part modeling a truncated-at-zero distribution, such as the P, NB, etc. This modeling strategy allows for differentiation between the process generating the zeroes, and that generating all other count values. Although the DMFT/DMFS indices has withstood the test of time as the prime endpoints of caries assessment, it comes with its own set of limitations. Aggregative in nature, it provides a summary caries index for the whole mouth, ignoring the details at the tooth, or tooth-surface level. Motivated by data generated from a clinical study of a Type-2 diabetic population [11], the objective of this paper is to conduct caries assessment at the ‘tooth-level’ considering the tooth-level DMFS count as the response for each tooth clustered within a subject and quantify its association with important covariables also collected in the study. Note, the tooth-level DMFS responses will now vary from 0 to 4, or 5, depending on the tooth-location, as in (Figure 1). For the anterior teeth (incisors and canines), each tooth contributes 4 surfaces, while the non-anterior ones (pre-molars and molars) contribute 5 surfaces per tooth. Here, we follow the DM5S convention [12], i.e., if a tooth is missing, we consider all the surfaces to be missing. Henceforth, any reference to DMFS in this manuscript means ‘tooth-level’ DMFS.
Samples
All 282 participants in this dataset were part of a study conducted at the Medical University of South Carolina (MUSC), located at Charleston, SC, on assessing the periodontal health status of Type-2 diabetic Gullah-speaking African-Americans [11]. The Gullah are a direct descendant population of rice plantation enslaved Africans from West Africa [13]. Gullah refers to several things: language, people, and a culture. The study received IRB approval at the Medical University of South Carolina (HR # 10637). Patients were recruited as part of Project SUGAR [14] via advertisements at health fairs, medical and dental clinics, in and around the Charleston, SC area.
Measurements
Our response variable is the DMFS count per tooth, with a total of 28 teeth per individual (excluding the third molars) for each of the 282 participants within this dataset. The upper bound for each subject’s DMFS was considered to be 4 or 5, depending on the tooth’s location within the mouth. In addition, the socio-demographic and behavioral variables that were collected were Age at inspection (mean=55.5, variance=10.9), Gender (74.4% female), Smoking Status (30.0% current/previous smokers), and an indicator variable for HbA1c status, coded as 1 for subjects with HbA1c 6.5% defined as poorly controlled Type-2 diabetes, T2D (61.6% of individuals) by the American Diabetes Association, and 0 for well-controlled. Among the covariates, we also include indicators of tooth-types, i.e., if the tooth is a canine, premolar, or molar (considering incisor as the baseline). Note, the current work is a secondary statistical analysis looking only on the caries responses among the Gullah subjects in a dataset that was generated primarily to study the incidence of T2D, and its association to periodontal disease.
Statistical Analysis
From (Figure 1), our data appears to be distributed as a
Binomial, with an upper bound of 5, and likely contains an excess
of zeroes from the healthy teeth. Because our motivating dataset
is somewhat homogenous (all Type-2 diabetic, Gullah-speaking
African Americans, with no controls), the zeroes are considered to
be ‘sampling’ zeroes. With our goal for better understanding the
effectiveness of various modeling strategies for the DMFS responses
in the context of ‘excess’ sampling zeroes and/or overdispersion,
we will look at three separate models, viz., the Binomial (B), Beta-
Binomial (BB), and 2-part Hurdle-Binomial (HB) models. More
details on these models appear in [15]. From (Figure 1), we can
expect the analysis using the basic B model (with random effects
accounting for clustering) will perform worse as compared to the
BB and the HB models, because the B model cannot handle excess
zeros, or overdispersion. What is interesting is to quantify the
extent to which our BB and HB models provide a better fit to our
data, and which modeling strategy appears to provide a superior fit.
Furthermore, the covariate- response relationship for determining
caries status will be conducted by connecting the important
covariables to the tooth-level DMFS response via. suitable link
functions.
We begin by examining the statistical background of the BB
model. Let Y be a random variable that denotes a bounded count
response (i.e., the DMFS), with y being our observed value of the
count. Define to be the probability mass function for
Y, corresponding to the ith individual and the jth tooth. In our Beta-
Binomial specification, our distribution is defined by
where θij is drawn from the Beta distribution, i.e.,
. Next,
one parameterizes aij and bij as using aij = μij * φ and bij = (1−μ)* φ ,where φ
is an unknown, but estimable, dispersion parameter and μij = E (Yij).
The data covariates are then connected to the true response Y via
a link function (e.g., logit) on μij. For the B model, one can simply
connect the covariates via a logit link on θij . For our HB model,
we consider the data generated from two independent processes,
with the modeling of zeros being done initially, followed by the
truncated-at-zero Binomial distribution for all counts greater than
zero. The HB distribution is generally defined aswhere,
p is the probability of a zero, and 1 − pij is the probability
of crossing the Hurdle, or the probability of observing a nonzero
count, and f(yij) is the distribution function of the Binomial
specification as described earlier. Once again, similar to the BB
model, we can connect the covariates to μij
through a logit link. We can estimate pij from the dataset or connected to the covariates
via. a logit link function. Note, in all these models, we will consider
a subject-level random effect term Ui to control for the effect of
heterogeneity due to clustered observations for subject i. Ui now
follows a normal distribution with an unknown (but estimable)
variance, i.e., . The U behaves as a subject-specific intercept term for each subject i. Due to the specific data structure, and the
lack of inbuilt distributions (such as the BB, or the H models), we
resort to the power of the NLMIXED procedure in SAS [16] for all our
data analysis. The NLMIXED procedure allows for the maximization
of any distribution for which the log- likelihood can be defined
using the ‘general ()’ function within the MODEL statement. The
immense flexibility provided via this function allows for the 2-part
or mixture modeling, such as the H models, as well as more intricate
distributions, such as the Beta-Binomial to be fit and maximum
likelihood (ML) parameter estimates to be obtained. The NLMIXED
code for this analysis can be requested from the first author.
Model comparisons
To evaluate the effectiveness of the competing models, and to determine which one provides the best fit to the DMFS dataset, we compare the deviance and the AIC/BIC criterion (where a lower value indicates a superior fit). From (Figure 1), it is apparent that there are a large amount of excess zero values, further supporting the use of an HB (or 2-part) model. Additionally, the presence of a significant amount of DMFS values at 4 and 5 might be suggestive of overdispersion, which can be tackled via the BB model. From (Table 1), it is clear that the B model provides the worst fit among the 3 models in terms of all the three criteria. The values are significantly greater than the HB model, and almost twice that of the BB model. The poor fit presented by the B model is not surprising. A closer look into the DMFS histogram in (Figure 1) reveal that the fundamental properties of the Binomial distribution are violated, and the real data has far greater number of zeroes, as well as the extremes (4 and 5) as expected under the B model. Even, the inclusion of random effects (supposed to accommodate model heterogeneity) failed to provide any improvement to the fit statistics. From (Table 1), we conclude that the overall model fit provided by the BB model (with the subject- specific random effect) is the best across all 3 criteria. Although the BB model does not address the source and extent of the excess zeroes as much as the HB model, the BB model allows for the additional heterogeneity via the dispersion parameter, coupled with the inclusion of the subject-specific random effects. The HB model with bivariate random effects having two randomeffect terms, one from the modeling of pij and the other from θij ) provided significantly better model fit, compared to the B model. The preference of the BB, or the HB model over the B model stems directly from data overdispersion, an issue which the B model is unable to accommodate. The overall superior fit provided by the BB model compared to the HB model can be attributed to some extent to the over-dispersed counts at both the lower ( niii = 0) and upper ( niii = 4, 5) levels within our data. Although the HB model provides an adequate method to capture the zero count values presented, it does not appear to adequately handle the large number of counts at the 4 and 5 level, as where the BB model capable of accounting for overdispersion as a whole does not identify a specific count value as the source of the overdispersion.
Parameter Estimates and Interpretation
(Table 2) reports the parameter estimates, their corresponding standard errors and 95% confidence intervals (CI) resulting from the model fit for the best fitting models under each of the 3 model classes: B, BB and HB. These are the B (with random effect), BB (with random effects), and the HB (with bivariate random effects). For the B and the BB models, the estimates for the covariates can be interpreted as the increase (or decrease) in the odds of the mean probability of observing an additional DMFS surface within a tooth, controlling for all other covariates and subject-level random effects, at the 0.05 level. However, interpretation of covariates in the HB model are more complex. There, one would have 2 sets of covariates; one that would explain association of observing a 0-count, while the other explains the association, conditional on crossing the hurdle of having at least one caries count. Although the BB model is the clear winner, we summarize our findings regarding the covariate associations and interpretations from these 3 models below.
Table 2: Parameter estimates, standard errors (in parenthesis), and 95% Confidence Intervals for the 3 competing models. Est. = Estimates, SE = Standard Errors, LCL = Lower 95% confidence limit, and UCL = Upper 95% confidence limit. H denotes the Binary regression (before crossing the Hurdle), while TB denotes the Truncated Binomial regression fitting for the HB model. Note, for the B and BB models, there is no truncation at 0; parameter estimates need to be interpreted accordingly.
Binomial (with RE)
With the inclusion of the subject level covariates age, gender, smoking and HbA1c, and tooth- level covariates of tooth-type, we observe significant association with respect to both age (p<0.05) and tooth types (p<0.05). Covariates that are not significant are not interpreted. From the resulting model estimates, for every 1-year increase in age we would expect an approximate increase in the mean probability of observing an additional DMFS of 4.5%. Additionally, the expected probability of an additional DMFS was 84.4%, 96.6%, and 93.2% lower for premolars, canines, and incisors, compared to molars. The estimated variance component associated with our random effect Ui is significantly greater than 0 (p<0.05) and quantifies the overall subject-level variability present within our dataset.
Beta-Binomial (with RE)
Similar to the B model above, the resulting parameter estimates
for the BB model suggest a significant association between age, and
tooth-location. Based on our estimates, for every 1-year increase
in age, we would expect to see an increase in the mean probability
of observing an additional DMFS of 4.9%. For tooth location, we
expect a decrease of 84.6%, 96.9%, and 94.2% in the expected
probability of an additional DMFS for premolars, canines, and
incisors respectively, compared to molars. Based on the significance
of our subject specific random effect estimate, we conclude that a
significant amount of variability within our model can be attributed
at the subject level. The estimate of φ (the dispersion parameter)
is 0.506, which estimates the model overdispersion to
be 2.99 for (for ij n =4), and 3.66 for (for ij n = 5). These values are
indicative of substantial overdispersion.
Hurdle-Binomial (with bivariate RE)
Interpretation of the HB model is two-part. With respect to
the likelihood of observing a 0-count value (i.e., failing to cross our
hurdle), a significant association was observed with respect to age
and tooth type. The model suggests that for every 1-year increase
in age, subjects are 3.7% less likely of observing a zero count.
However, compared to molars, the premolars, canines, and incisors
were significantly more likely 995.5%, 6,386.7% and 3,786.1%
more likely to observe a 0-count value. This clearly implies that
the molars have a significantly low probability of remaining caries
free, compared to the other tooth types. molars. With respect to the
truncated binomial regression (which conditions on the fact that a
non-zero carious count is observed), a significant association was observed with respect to age and tooth type. An expected increase in
the likelihood of an additional DMFS of 5% for every 1-year increase
in age is observed, and premolars, canines, and incisors exhibit an
expected decrease in the likelihood of observing an additional DMFS
of 66.1%, 76.8%, and 44.8% respectively, compared to the molars.
From the estimates of and σ (the variance components and
correlation parameter of the bivariate normal density assumed for
the bivariate random effects); we conclude that a large amount of
the model variability can be attributed at the subject level.
The analysis of clustered count data with finite upper bounds that exhibits overdispersion, and excess zeros remains a complex statistical problem. This paper aims to illustrate the effectiveness of the B, BB, and HB models in modeling these types of data through application to a DMFS data on dental caries. Although initial raw data plots and the underlying disease progression mechanism pointed towards a Hurdle (2-part) model specification, empirical results contradicted our prior belief, and chose the BB model as the model of best fit. It is apparent that the preponderance of zeroes in any dataset does not immediately qualify for an “excesszero” modeling, via. the Hurdle or the ZI models as the case might be. Careful considerations should be made with respect to the appropriate model choice. The methodological issues discussed here should provide some guidance in the model building procedure. The authors recommend choosing the best model (from an a priori chosen class of models) through available goodness- offit statistics, such as the deviance, AIC, BIC, etc. It is also important to note that the a priori chosen class of models to be fitted may vary with the dataset and its characteristics under consideration. For example, for this GAAD dataset, a higher proportion of carious molars is reflected in the shape of the histograms in (Figure 1), leading to possible binomial overdispersion better captured by the BB specification. The histogram features are expected to vary for a different caries data. This would lead to a new a priori chosen class of models for fitting. In a clinical context, this study throws new light into caries assessments. The rate of caries progression is not homogenous, and different regions of the mouth are susceptible to different degrees of carious lesions (such as, molars can be different than incisors). Our ‘tooth-level’ DMFS investigation can provide inference and prediction for each tooth at various locations inside the mouth, which are not possible using the popular full-mouth DMFT/DMFS measures. Our current motivating Gullah dataset records tooth-level DMFS counts cross-sectionally. In this context, dental clinical trials assessing caries progression can generate clustered-longitudinal tooth-level DMFS counts, and our proposals can be modified to handle longitudinal count data to evaluate the effect of possible interventions, in addition to modeling the entire longitudinal profile of each tooth from an arbitrary subject. This is a subject of future research and will be considered elsewhere, including large U.S. dental healthcare systems with longitudinal data repositories.
The authors thank the Center for Oral Health Research at the Medical University of South Carolina for providing the motivating data, and the context of this work. The work of Drs. Bandyopadhyay and Russell are supported in part by grants R01DE024984 and R01DE029963 from the National Institutes of Health.
Bio chemistry
University of Texas Medical Branch, USADepartment of Criminal Justice
Liberty University, USADepartment of Psychiatry
University of Kentucky, USADepartment of Medicine
Gally International Biomedical Research & Consulting LLC, USADepartment of Urbanisation and Agricultural
Montreal university, USAOral & Maxillofacial Pathology
New York University, USAGastroenterology and Hepatology
University of Alabama, UKDepartment of Medicine
Universities of Bradford, UKOncology
Circulogene Theranostics, EnglandRadiation Chemistry
National University of Mexico, USAAnalytical Chemistry
Wentworth Institute of Technology, USAMinimally Invasive Surgery
Mercer University school of Medicine, USAPediatric Dentistry
University of Athens , GreeceThe annual scholar awards from Lupine Publishers honor a selected number Read More...