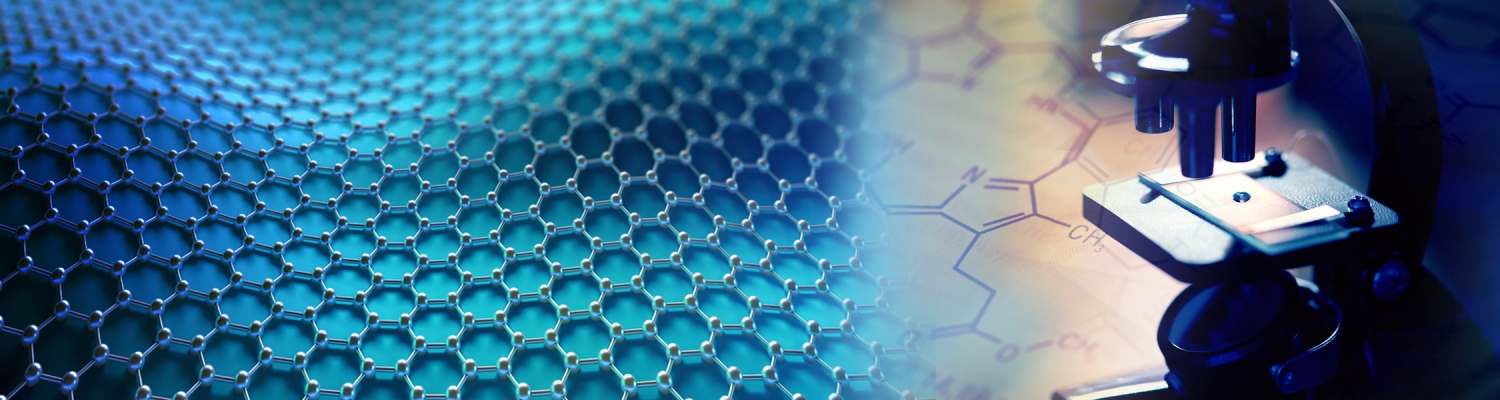
ISSN: 2641-6921
Wong CH1,2*, Raymond PH4, Wu H5, Lei X3 and Zatsepin AF3
Received:April 12, 2023; Published: May 10, 2023
*Corresponding author:Wong CH, Department of Industrial and Systems Engineering, The Hong Kong Polytechnic University, Hong Kong, China
DOI: 10.32474/MAMS.2023.05.000208
For the superconductors suffering from phase fluctuations, finite electrical resistance exists below the pairing temperature Tc unless it undergoes Berezinskii-Kosterlitz-Thouless (BKT) transition where vortices and anti-vortices form pairs a particular temperature TBKT. As the distribution of vortices carries valuable information to understand the vortex-melting in superconductors, the Tc and TBKT must be identified in heavily overlapped heat capacity anomalies. To avoid misjudging TBKT, we test whether or not the Google Net model can separate the overlapped anomalies without losing the primary information about the phase transitions, and test if it predicts the Tc and TBKT in a new system accurately. Our work opens a path for the Google Net model to enter the world of superconductivity as an artificial-intelligence tool.
Keywords: Superconductors; Deep learning; Monte Carlo simulations
To vanish the electrical resistance of superconductors, the phase and amplitude of the superconducting parameters must be ordered [1,2]. The phase fluctuations in low-dimensional superconductors and high-temperature bulk superconductors suppress the formation of a long-range ordered state even if the temperature is below the Tc [2-15]. The phase stability can be achieved by undergoing the Berezinski–Kosterlitz–Thouless (BKT) transition [3-5] at a lower critical temperature TBKT, where a quasi-long range superconducting order is created. Despite the van Hove singularities [6] in the electronic density of states of 1D superconductors would be advantageous to produce a high Tc phenomenon, the superconducting phase slips at the 1D limit suppressing a long-range order at any finite temperature associated with a finite electrical resistance [7-11]. However, the disappearance of the phase fluctuations has been observed in the quasi-1D superconducting nanowires array under a transverse Josephson coupling J where a long-range-ordered superconducting state with zero resistance at finite temperature is obtainable [12-19].
In recent years, scientists aim at raising the TBKT closer to the Tc [12,14] through understanding the mechanism of vortex-antivortex pairing. The critical temperatures for the system with multiple phase transitions can be determined by searching the peaks of the heat capacity anomalies [4]. By inputting the experimental parameters (e.g., lattice parameters, electron concentration …etc) into the XY model, the BKT transition and superconducting phase transition [4-20] can be generated where the degree of overlap is comparable to the experimental observations [11-20]. By plotting the distribution of phase vortices in the XY model, it unmasks the mechanism of vortex melting in different superconductors. However, in high-temperature bulk superconductors like Nb3Sn, the heat capacity anomalies at the Tc and the TBKT are heavily overlapped where the difference between these two-phase transition temperatures is ~0.2K only. When the experimental parameter of the Nb3Sn superconductor is imported to the XY model, the almost completely overlapped anomalies in the XY model makes it difficult to identify the value of TBKT accurately [15] even if advanced curve-fitting techniques are applied. If the distribution of phase vortices is misjudged at the wrong BKT transition temperature, it impedes the search for the mechanism behind the superconducting vortex-antivortex pairing. To solve this issue, a phase transition detector without showing overlapping phase transitions on the temperature domain is needed. The Google Net designed by the research teams at Google with the collaboration of several universities has been a forefront computer vision algorithm since 2014. [21] The Google Net model contains 1×1 convolutions in the middle of the architecture and global average pooling that establishes a deeper architecture of 22 layers deep with low computational cost [22,23]. Before we study the significantly overlapped transitions in the Nb3Sn superconductors [15] we test whether the Google Net-assisted phase transition detector can separate the moderately overlapped transitions in the parallel 1D superconducting nanowires laterally coupled by Josephson interaction J as a preliminary investigation.
The Monte Carlo method with the Metropolis algorithm is used to simulate the standard 8-state XY model (q = 8) on a square lattice, where the Josephson interaction J is spatial independent along the sample surface [4,7,12]. The phase maps as a series of temperature at equilibrium state are obtained. Then the phase maps are converted into the JPEG format for image classification. To identify the Tc at which two electrons form a Cooper pair (CP) [7] two thousand phase-maps with the size of 66 x 66 are simulated at the dimensionless Tc under the condition of average J = |Jsc sin (δʘ)| = 1, which is named the “stock data (CP)”. The Jsc is the magnitude of Josephson energy and δʘ refers to the phase difference during the tunneling process. To locate the TBKT at which the vortex and anti-vortex form a pair [4,20], two thousand phase-maps with the size of 66 x 66 are generated at the dimensionless TBKT under the condition of J = 1, which is named as the “stock data (BKT)”. Then we create the “test data (q = 8, J = 1)” and the “test data (q = 8, J = 0.8)” where two thousand 66 x 66 phase-maps are generated at each temperature, respectively. The image classification is based on the GoogLeNet model under the Convolutional Neural Network (CNN) [21,22]. The image database is split into training data (70%) and validation data (30%). If the Google Net model can recognize the phase maps accurately, the validation accuracy of phase maps is close to 100%. To check whether the sample is located at the phase transition temperatures or not, the likelihood of the phase transitions, i.e. LH factor (CP) and LH factor (BKT), are defined as (100% - validation accuracy) / 50%. When the LH factor closes to 1, the material is expected to undergo the phase transition.
Figure 1 shows the thermal fluctuations which are generated by the 8-state XY model with J = 1 and J = 0.8. After a careful curve-fitting process, we get the TBKT = 0.34 and the Tс = 1.13 for J = 1 in Figure 1a. Similarly, we get the TBKT = 0.32 and the Tс = 0.85 for J = 0.8 in Figure 1b. This verifies that the values of TBKT and Tс depend on the Josephson interaction J. The two thousand phase-maps simulated in the conditions of J = 1 and Tc = 1.13 are grouped as the “stock data (CP)”. Meanwhile, the two thousand phase maps in the “stock data (BKT)” are created in the conditions of J = 1 and TBKT = 0.34. The phase maps are plotted in Figure 2, respectively. The direction of phase is displayed in terms of a color-plot which is advantageous to image classification. In the 8-state XY model, the direction of superconducting phase at 0, 45, 90, 135, 180, 225, 270, 315 degrees refers to the integer 0, 1, 2, 3, 4, 5, 6, 7 in the color bar, respectively. Figure 1: Alt Text [21 words]: The black lines are the heat capacity data. The red curve is the smoothed data. Two heat capacity anomalies are observed. Figure 2 Alt Text: Four figures are shown. The distribution of grid color is more chaotic in the ascending order of (2a) < (2b) < (2c) < (2d) [21 words] According to Figure 3, the Google Net-assisted phase transition detector distinguishes the Tc and the TBKT on the same domain very clearly. Although the traditional method [4] in Figure 1a shows that the BKT transition occurs at the TBKT ~ 0.3, Figure 3a shows that the LH factor (CP) remains zero for T < 0.5. Zero LH factor (BKT or CP) means that undergoing the phase transition is not possible. For J = 1, the Google Net-assisted phase transition detector shows that the Tc is 1.1 with the evidence of the LH factor (CP) = 1. On the other hand, by replacing the “stock data (CP)” with the “stock data (BKT)” emerges the BKT transition at TBKT ~ 0.3 as shown in Figure 3b, where the LH factor (BKT) = 1. The LH factor (BKT) returns to zero for T > 0.6 even though the superconducting phase transition occurs at Tc ~ 1. As the stock data and the test data in Figure 3 are computed by setting the J = 1 in the XY model [4] we proceed to examine the accuracy of the Google Net-assisted phase transition detector if the Josephson interaction in the stock data and the test data are not identical. Figure 4 demonstrates that the Google Net model [21,22] is capable of learning the features from the phase-maps in the J = 1 system and then interpreting the unknown phase transition temperatures in the ‘unseen’ systems (J ≠ 1).
Figure 2: The phase-maps for J = 1 at different temperatures. (a) The phase-map below the TBKT. (b) The phase-map at the TBKT. (c) The phase-map at the Tc. (d) The phase-map above the Tc.
Figure 3: The LH factors as a function of temperatures. The stock data (CP), the stock data (BKT) and the test data are run by assigning the J = 1 in the XY model (a) The LH factor (CP) refers to the likelihood of forming the superconducting phase transition. (b) The LH factor (BKT) refers to the likelihood of undergoing the BKT transition.
Figure 4: The LH factors as a series of temperatures. The stock data (CP), the stock data (BKT) are run by assigning the J = 1 in the XY model. The test data are prepared by setting the J = 0.8 in the XY model. (a) The LH factor (CP) of 1 corresponds to the superconducting phase transition temperature. (b) The LH factor (BKT) of 1 refers to the BKT transition temperature.
The prediction of the Google Net model in the J = 0.8 system is also accurate. Although Figure 1b illustrates the BKT transition at T ~ 0.3, Figure 4 confirms that the GoogLeNet-assisted phase transition detector can distinguish the Tc and the TBKT effectively without overlapping. After the J is reduced to 0.8 in the “test data”, Figure 4a shows that the LH factor (CP) is the highest at Tc = 0.83 where the superconducting phase transition takes place. No BKT transition is observed in Figure 4a. Again, in Figure 4b, changing the “stock data (CP)” to the “stock data (BKT)” emerges the BKT transition at T ~ 0.3 and the superconducting phase transition at T ~ 1 is disappeared. The LH factor (BKT) becomes zero for T > 0.7 in Figure 4b. Figure 3: Alt Text: (3a) A peak of the bump is located at ~T=1. (3b) A peak of the bump is located at ~T=0.4. [20 words] Figure 4: Alt Text: (4a) A peak of the bump is located at ~T=0.9. (4b) A peak of the bump is located at ~T=0.4 [20 words]. When we draw a parallel between Figure 1, Figure 3 and Figure 4, the phase transition temperatures probed by the GoogLeNet model [21,22] are almost identical to the traditional method of heat capacity measurement [4,12,20]. However, there is a big advantage of using the GoogLeNet-assisted phase transition detector: the superconducting phase transition and the BKT transition can be observed separately. If the traditional method is used to probe the phase transition temperature [4] these two heat capacity anomalies emerge on the same temperature domain in Figure 1, which requires advanced numerical methods [12-15] to distinguish the TBKT and the Tс. Without the advanced curve fitting techniques, one may argue that there are three heat capacity anomalies at T ~ 0.3, T ~ 1 and T ~ 1.7 in Figure 1a. After we fit the curves carefully with help of the scientific knowledge and numerical mathematics [12-15] we can figure out that the peaks at T ~ 1.7 do not refer to the superconducting phase transition temperature. In contrast, probing the superconducting transition temperature via the use of the LH factor can avoid this problem because the non-zero LH factor (CP) and LH factor (BKT) do not appear at the same temperature. Let us recall that a higher validation accuracy of the phase maps produces a lower LH factor. When the temperature is near the Tc or the TBKT, the phase-maps in the “stock data” and the “test data” look similar. Then it reduces the validation accuracy and raises the LH factor where we make use of this trend to locate the phase transition temperatures. The GoogLeNet-assisted phase transition detector is accurate as shown in Figure 3. It is reasonable because the Josephson interactions in the “stock data” and the “test data” are the same. As the GoogLeNet model has learnt the features from the stock data at J =1, it interprets the phase map in the ‘unseen’ system (J = 0.8) successfully, because the distribution of phases at the Tc (or TBKT) shares the same nature regardless of the value of J. We used a FUJITSU computer equipped with Intel(R) Core (TM) i7-3632QM CPU at 2.2GHz to train the phase transition detector that requires 25 minutes only. With this reasonable computational cost, it is possible to predict the phase transitions by using the artificial intelligence assisted XY model. As the Google Net-assisted XY model shows great success in separating the moderately overlapped phase transition, it gives hope to distinguish the Tc and the TBKT in a heavily overlapped transition such as Nb3Sn superconductors and is possibly applicable to other superconductors suffering from the same issue.
The author(s) declare no competing interests.
We appreciate the Research Institute for Advanced Manufacturing and the Industrial Centre at The Hong Kong Polytechnic University to support this project. This research project was sponsored by (University Grants Committee – The Hong Kong Polytechnic University) with grant number (1-45-37-BD5C).
Data available on request from the authors
Bio chemistry
University of Texas Medical Branch, USADepartment of Criminal Justice
Liberty University, USADepartment of Psychiatry
University of Kentucky, USADepartment of Medicine
Gally International Biomedical Research & Consulting LLC, USADepartment of Urbanisation and Agricultural
Montreal university, USAOral & Maxillofacial Pathology
New York University, USAGastroenterology and Hepatology
University of Alabama, UKDepartment of Medicine
Universities of Bradford, UKOncology
Circulogene Theranostics, EnglandRadiation Chemistry
National University of Mexico, USAAnalytical Chemistry
Wentworth Institute of Technology, USAMinimally Invasive Surgery
Mercer University school of Medicine, USAPediatric Dentistry
University of Athens , GreeceThe annual scholar awards from Lupine Publishers honor a selected number Read More...