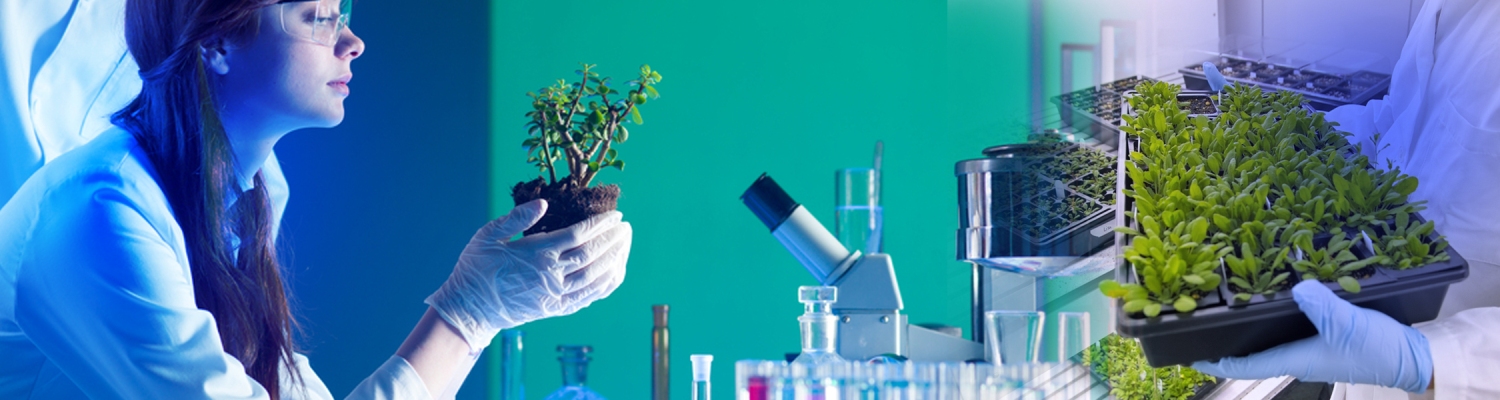
ISSN: 2641-6794
Akshey Bhargava1*, Megha TS2 and SheetalKamble3
Received: February 04, 2021 Published: February 24, 2021
Corresponding author: Akshey Bhargava, Ex. Rajasthan Pollution Control Board, CEPT University, India
DOI: 10.32474/OAJESS.2021.06.000229
Air pollution has been termed as a democratizing force, but it is far from that, as it propagates existing environmental injustices. Industrial development and rapid urbanization have adversely affected urban air quality due to industrial and vehicular emissions. Air pollution exposure has shown to affect cognitive development in children, slow lung development and has resulted in high levels of mortality from respiratory infections. The elderly is more likely to develop cardiac illnesses and chronic respiratory as a result of long-term exposure and are more susceptible to strokes and heart attacks during episodic high pollution events. Thermal Power Plant has been a major source of air pollution for so long. Increasing health problems has made particulate matter to give special attention by control technology and framing regulations. Suspended particulate matter (SPM) is considered to be the main pollutant emitted by the power station. Atmospheric dispersion pollution modelling is of great and actual concern in the scientific international community. Many dispersion models have been developed and used to estimate the downwind ambient concentration of air pollutants from sources such as industrial plants, vehicular traffic, or accidental chemical release. Among them, Gaussian model is perhaps the most commonly used model type. It is often used to predict the dispersion of air pollution plumes originated from ground-level or elevated sources. An attempt has been made in the present paper by the authors to discuss such models with a view to select a particular model that can be used for a particular area or application. An effort has also been made to predict SPM concentrations from a coal based thermal power plant at various receptor points using model of Gaussian dispersion.
Keywords: Suspended particulate matter (SPM); Thermal power plant; Air dispersion model; Gaussian approach; Dispersion coefficients; Predictions; Urbanization; Gaussian model; Atmospheric dispersion modelling; Meteorology; Mitigation; Air pollution; Turbulent fluxes; Atmosphere; Estimated wind velocities
The dispersion of air pollution both in urban areas and open
spaces is becoming of great concern in the scientific community. The
concern for air quality has been an issue with Indian government
for the past few years. Growing urbanization along with industrial
and vehicular pollution is leading to environmental degradation
faster than in other countries. A thriving industrial base and rapid
economic growth–about 5% a year–account for much of the severe
pollution, which costs India an estimated $9.7 billion a year in
environmental damage. In last decades, the normal levels of air
pollution have increased [1] and many countries have started to
focus to develop monitoring systems for air pollution. Air quality
models are able to predict the pollutant gases or aerosol trajectories
in atmosphere. The evaluation and calibration of dispersion
models is of a crucial importance, because their results often
influence decisions that have large public-health and economic
consequences. Obviously, there are different types of models and
their performances depend on many variables. The classification of
these models may refer about the source type (point source, line
source, and area source), the adopted scale (large or small scale),
the input type (deterministic models and stochastic models), the
dynamic conditions (steady or unsteady state), and the pollutant
sources (gases or particles). Among them, Gaussian model is
perhaps the most commonly used model type. These are widely
used in atmospheric dispersion modelling, usually in regulatory purposes because of their easy implementation and their near realtime
responds. They generally are used in large scale applications
[2]. Suspended particulate matter (SPM) levels in the air have been
found to be at critical levels in urban cities, especially in residential
areas, in India. The ambient levels of SPM in most of the urban
areas of India are above 150mg/m3, which far exceeds the WHO
standards of 50mg/m3 [3]. The coal-based thermal power plants,
constitute 62.3% of electric power generation, are considered to
be one of the chief industrial emitters of SPM in India. Increasing
reliance on coal as the main fossil fuel in thermal power generation
has led to numerous environmental problems [4]. Table 1 gives the
share of coal and other fuels in electric generation along with the
amount of SPM emitted per kilogram of fuels used.
Air pollution modelling is a numerical tool used to describe the causal relationship between emissions, meteorology, atmospheric concentrations, deposition, and other factors. This tool can give a complete deterministic description of the air quality problem, including an analysis of factors and causes (emission sources, meteorological processes, and physical and chemical changes), and some guidance on the implementation of mitigation measures [5]. One of the main challenges of atmospheric dispersion modelling is to develop models and software that can provide numerical predictions in an accurate and computationally efficient way. The concentrations of substances in the atmosphere are determined by 1) transport, 2) diffusion, 3) chemical transformation, and 4) ground deposition. The study of diffusion (turbulent motion) is more recent [5]. Chemical species such as NOx, SO2, which have longer lifetimes (hours-days) can have wider impact zones [6]. Therefore, in such cases regional or continental modelling approaches are needed. For simulating the dispersion of air pollutants, various modelling approaches have been developed. The application of air dispersion models [7] are quite wide in as much as that it is effectively used for urban planning, industrial estate planning, industrial zoning, sitting of industrial project and overall special planning from environmental point of view. Modelling can be used to analyze actual or potential accidents that release contaminants to the atmosphere. Other applications are as under:
1. Assessing compliance of emissions with air quality guidelines,
criteria, and standards
2. Planning new facilities
3. Determining appropriate stack heights
4. Managing existing emissions
5. Designing ambient air monitoring networks
6. Identifying the main contributors to existing air pollution
problems
7. Evaluating policy and mitigation strategies (e.g., the effect of
emission standards) forecasting pollution episodes
8. Assessing the risks of and planning for the management of rare
events such as accidental hazardous substance releases
9. Estimating the influence of geophysical factors on dispersion
(e.g., terrain elevation, presence of water bodies and land use)
10. Running ‘numerical laboratories’ for scientific research
involving experiments that would otherwise be too costly in
the real world (e.g., tracking accidental hazardous substance
releases)
11. Saving cost and time over monitoring – modelling costs are
a fraction of monitoring costs and a simulation of annual or
multi-year periods may only take a few weeks to assess.
Air pollution models can be categorized into three generic classes: deterministic approach, statistical models, and physical models. Deterministic models basically deal with different types of numerical approximations (for example finite difference and finite techniques) in the solution of the partial equations representing the relevant physical process of atmospheric dispersion. The deterministic model is most suitable for long-term planning decisions. In contrast to the deterministic model, the statistical one calculates ambient air concentrations using an empirical established statistical relationship between meteorological and other parameters on the other hand [8]. The statistical model is very useful for short-term forecast of concentrations. In physical models, a real process is simulated on a smaller scale in the laboratory by a physical experiment, which models the important features of the original process being studied. The most important advantage of physical models is that the scale-model geometry, as well as flow speed and other essential variables can be easily changed and controlled [8].
Most of the deterministic model use or are equivalent to using solutions of the diffusion equation. The diffusion equation is based on the principle of conservation of mass. The turbulent fluxes of material are expressed by the gradient relationship, i.e., it is assumed that the turbulent flux is proportional to the gradient of concentration and the proportionality ‘constant’ is called the diffusion coefficient. The diffusion equation reads as follows:
The symbols have following measuring:
c = the time – averaged concentration
x, y, z = the Cartesian coordinates
u, v, w = the components of the time-averaged wind vector
kx, kY, kZ = the diffusion coefficient in the corresponding
direction
The boundary condition at the earth’s surface has to be stated
and it is often assumed that the diffusing material (gases) is not
absorbed by the ground, i.e., the material is ‘reflected’ into the
atmosphere. The other extreme case that all material reaching
the surface is absorbed, is not appropriate, at least not for gases.
Partial reflection of the material at the ground is the most realistic
case, but it involves the statement of another parameter, which is
usually called the deposited velocity. Often a boundary condition
for the upper boundary of the diffusion volume is stated, especially
in case of an inversion [8]. In that case, too, the total reflection
assumption at the upper boundary is used. The models calculate
the concentration at one receptor point from one source. If more
than one source is present, then the contributions from each source
at the receptor point are summed.
Gaussian plume model
The greatest advantage of Gaussian Plume models is that
they have an extremely fast, almost immediate response time.
Their calculation is based only on solving a single formula for
every receptor point, and the model’s computational cost mainly
consists of meteorological data pre-processing and turbulence
parameterization. Depending on the complexity of these submodules,
the model’s runtime can be extremely reduced enabling
its application in real-time and near real-time decision support
software [9]. Gaussian dispersion models have become a uniquely
efficient tool of air quality management for the past decades,
especially in the early years when high performance computers had
an unreachable price for environmental protection organizations
and authorities. Their fast responds depend basically on several
assumptions that make them useful for just some applications. The
main important assumptions are:
a) The emission rate of the source is constant.
b) Dispersion (diffusion) is negligible in the downwind direction.
c) Horizontal meteorological conditions are homogeneous over
the space modelled.
1. For each step modelled:
a. Wind speed is constant.
b. Wind direction is constant.
c. Temperature is constant.
d. Mixing height is constant.
e. No wind shear in the horizontal or vertical plane.
f. The pollutants are non-reactive gases or aerosol.
g. The plume is reflected at the surface with no deposition or
reaction with the surface.
h. The dispersion in the crosswind and vertical direction takes
the form of Gaussian distributions.
The sources types may be different: point source, volume source,
area source, open pit and flare. Usually, it is possible to implement
Gaussian models for more than one source and as assumption it is
considered the Superposition principle.
An effort has been made in the present paper to develop air dispersion model by using Gaussian distribution approach. While developing this model, source strength of SPM based on composition of fuel has been estimated along with meteorological conditions were analyzed on Annual and seasonal basis to form a part of input data in the model. Similarly, plume rise from 10 model equations were estimated and the average value of the plume rise was considered in the present model. The atmospheric stability was also analyzed for making use in this model. The ground level concentrations of SPM were estimated under all stability conditions starting from A to till category F. An isopleths were then prepared using surfer9 software. The predictions were done up to a distance of 120 km from the Gandhinagar thermal power plant. The vertical and horizontal dispersion coefficients were estimated using following equations.
By using above equations and assuming constants as referred in above Tables 1 & 2, the values so obtained for σy and σz at various distances are as under (Tables 3 & 4). Subsequent to estimation of σy and σz under all atmospheric stability conditions, the wind velocity at stack heights of Gandhinagar thermal power plant were estimated using power law, the equation of which is as under.
Where, μ1 and μ2 are wind speeds at height Z1 and Z2
respectively.
n is an exponent, is a function of stability class as shown in
Table 5. Gandhinagar thermal power plant has five stacks attach to
different units of power production; the details of each stack are
as reflected in Table 6 below. The wind velocities at different stack
heights indicated in Table 6 above were estimated using power
law and values of exponent n referred in Table 4. The Average
Estimated wind velocities at different stack heights under different
stability conditions and directions for annual and different seasons
are given in the following Tables 7-10. By using above equations
and assuming constants as referred in above tables, the values so
obtained for σy& σz at various distances are as under: Subsequent
to estimation of σy&σz under all atmospheric stability conditions,
the wind velocity at stack heights of Gandhinagar thermal power
plant were estimated using power law , the equation of which is as
under.
μ1= μ2 (Z1/Z2) n,
Where, μ1, μ2 are wind speeds at height Z1 & Z2 respectively. n is an exponent, is a function of stability class and is shown in Table 5 below. Gandhinagar thermal power plant has five stacks attach to different units of power production, the details of each stack are as under as per field monitoring and analysis. The wind velocities at different stack heights indicated in table above were estimated using power law and values of exponent n referred in Table 6. The Average Estimated wind velocities at different stack heights under different stability conditions and directions for annual and different seasons are calculated and given in the following tables. The values of average wind velocities at stack heights under different directions were used in the model as one of the input for predicting Annual average, winter, summer & Monsoon ground level concentrations of SPM at different receptor points. Similarly, as an input to air dispersion model, the source strength of SPM was also estimated using different methods However, the source strength considered in the model is given in the following table for different stacks. Similarly, plume rise was estimated using different model equations. The plume rise considered in the model is given in the following table .Having all the above parameters as an input to air dispersion model, the predicted concentration of SPM was estimated on annual and seasonal basis. The isopleths were also drawn in respect of SPM for different seasons & on annual basis under all the stability conditions. On the basis of predicted values, the isopleths for Annual SPM concentration have been drawn for all the stability conditions and some of them are being given in the Figures 1-7. Similarly, an effort has also been made to analyze the maximum concentration of predicted Annual SPM values in each direction under different stability condition at a particular distance. Such estimation has been shown in Tables 13 & 14. Similarly, Table 15 showing maximum predicted concentrations of SPM on annual, winter, summer, and monsoon seasons under all atmospheric stability conditions (A - F). It would be evident from above table that maximum predicted concentrations of SPM occur during winter season (54.64 μg/m3) followed by annual, monsoon and least in summer (9.73 μg/m3) under atmospheric stability A. It would also tend to show that maximum concentrations occur under stability A and the concentration decreases with the shifting of atmospheric stabilities from A to F.
Table 14: Showing maximum concentration of Annual SPM at a distance under each stability category under different directions.
Table 15: Showing maximum predicted concentrations of SPN under all seasons and atmospheric stabilities.
An effort has also been made to analyze the maximum concentration of predicted Annual SPM values in each direction under different stability condition at a particular distance. The findings are such estimations are shown in Table 14. It would be seen from Table 13 that the maximum annual average SPM concentration is 13.66 μg/m3 at a distance of 2 km under atmospheric stability A in ESE direction, followed by11.05, 10.53,9.37,8.93 & 7.80 μg/ m3 in SSE,NNE,SSW,NNW,ENE directions respectively but all at a distance of 2 km. Similarly, under atmospheric stability B, maximum concentration of SPM is of the order of 148.55 μg/m3 at a distance of 4 km, followed by 84.88,12.49,10.33,9.30 & 9.25 μg/m3 in ENE,SSE,NNE, SSW,NNW, directions respectively but all at a distance of 4 km. Moreover, under atmospheric stability c, maximum concentration of SPM is of the order of 131.62 μg/m3 at a distance of 8 km, followed by 75.21, 11.06, 9.15, 8.24 & 8.20 μg/m3 in ENE ,SSE,NNE,SSW,NNW directions respectively but all at a distance of 8 km. Similarly, under atmospheric stability D, maximum concentration of SPM is of the order of 27.196 μg/m3 at a distance of 52 km, followed by 15.54, 2.28, 1.89, 1.70& 1.69 μg/m3 in ENE, SSE, NNE, SSW, NNW directions respectively but all at a distance of 52 km. However, under atmospheric stability E, maximum concentration of SPM is of the order of 10.13 μg/m3 at a distance of 120 km, followed by, 5.78, 0.85, 0.70, 0.634& 0.631 μg/m3 in ENE, SSE, NNE, SSW, NNW directions respectively but all at a distance of 120 km. Similarly, under atmospheric stability F, maximum concentration of SPM is of the order of 2.51 μg/m3 at a distance of 120 km, followed by , 1.43,0.21,0.175,0.157 & 0.156 μg/ m3 in ENE ,SSE,NNE,SSW,NNW directions respectively but all at a distance of 120 km. From the above, it tends to indicate that under stability A , the maximum concentration occurs at a distance of 2 km whereas, this distance Increased to 4 km under stability B, 8 km under stability c, 52 km under stability D and 120 km under stability E & F. This analysis tends to show that the distance increases with shifting of stability from A to F. i.e., from highly unstable to highly stable conditions. Similarly, an attempt was also made to predict the ground level concentrations of SPM under all stability conditions for the season winter, summer, and monsoon. The Isopleths were also prepared but not being shown in the present paper due to huge volume. However, the Maximum predicted concentration of SPM under all stability conditions for all the seasons are shown in Tables 14 & 15.
Air pollution is growing on a rapid pace, not only in India but globally. It is becoming an emerging issue threating the health of human beings and environment as a whole. Air dispersion modeling is an important decision-making tool to predict air quality from air pollution sources on a scale of time and space. Such models are mandatory in the process of Environmental Impact Assessment and Management Plans required for environmental clearances. However, lot of research needs to be done for developing a scientifically compatible air dispersion models having regard to prevailing local conditions, reliable source strength, meteorological factors etc. Such studies need to be done on scale of time and space. Data base should be developed to keep all such research at one place for the benefit of other researchers.
Bio chemistry
University of Texas Medical Branch, USADepartment of Criminal Justice
Liberty University, USADepartment of Psychiatry
University of Kentucky, USADepartment of Medicine
Gally International Biomedical Research & Consulting LLC, USADepartment of Urbanisation and Agricultural
Montreal university, USAOral & Maxillofacial Pathology
New York University, USAGastroenterology and Hepatology
University of Alabama, UKDepartment of Medicine
Universities of Bradford, UKOncology
Circulogene Theranostics, EnglandRadiation Chemistry
National University of Mexico, USAAnalytical Chemistry
Wentworth Institute of Technology, USAMinimally Invasive Surgery
Mercer University school of Medicine, USAPediatric Dentistry
University of Athens , GreeceThe annual scholar awards from Lupine Publishers honor a selected number Read More...