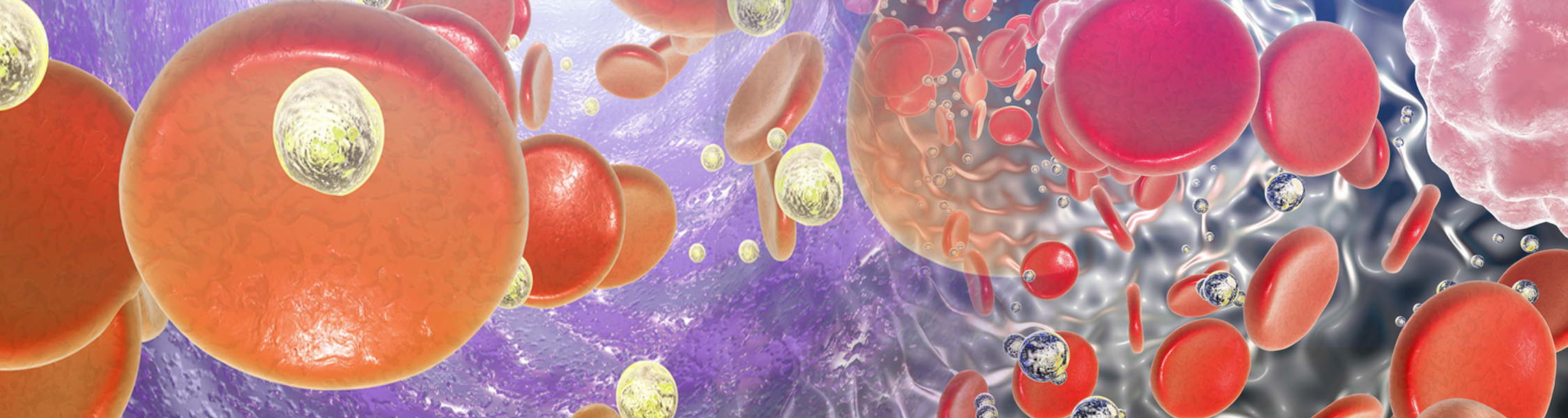
Gamal El Afandi1*, Mostafa Morsy2 and Hossam Ismael1,3
Received: December 02, 2022 Published: December 15, 2022
Corresponding author: Gamal El Afandi, College of Agriculture, Environment and Nutrition Sciences, Tuskegee University, Tuskegee, AL 36088, USA
DOI: 10.32474/JBRS.2022.02.000134
The wind is one of the main significant renewable energy resources not only for developing countries but also all over the globe. It could be used to overcome the increasing energy power demand in many countries, especially Egypt, Sudan, and South Sudan. In addition, it is a good source of clean energy that could be used to reduce air pollution in these countries. The main objective of this research is to evaluate the performance of the Weather Research and Forecasting (WRF) model to predict wind speed over Egypt, Sudan, and South Sudan. Nineteen weather stations were selected from the three countries for this purpose. Therefore, the statistical parameters of the Willmott Index of agreement (WI), Coefficient of Determination (R2), Normalized Root Mean Square Error (NRMSE), and Normalized Mean Bias Error (NMBE) were used in the evaluation processes. The results revealed that the WRF performance was high during autumn and winter in Egypt, Sudan, and South Sudan, while it was low during summer in all countries. In addition, strong correlations were found between measured and forecasted wind speeds for the selected days during all seasons. At the same time, WI and R2 values were approximately close to 1, while NRMSE and NMBE were approaching zero. Therefore, there is no significant difference between the real and forecasted wind speeds during all seasons. Overall results proved the capability of the model to predict wind speed over Egypt, Sudan, and South Sudan compared to measurements. Therefore, it may conclude that all countries could depend on the WRF model to predict wind speed in the past, present, and future with high accuracy.
Keywords: Wind speed; WRF model evaluation; statistical indicators
The wind is one of the most important determinants of the climatic conditions that shape weather systems over different regions of the earth’s surface [1]. Its first role and responsibility are transporting energy, momentum, and moisture horizontally and vertically in the atmosphere [2]. For example, the wind is carrying air masses and pollutants and disperses them from place to place on the Earth’s surface [3,4]. The earth’s surface heating and cooling because of an uneven geographical distribution of solar radiation incidence on the surface leads to pressure gradient forces, which in turn leads to winds. As a result, the evolution of horizontal pressure gradients, which are kept constant by the Coriolis force of the earth’s rotation, is the principal cause of air motion [5]. The atmosphere balances the uneven distribution of pressure and radiation (heating and cooling) over the surface of the Earth through winds. Wind resource assessment can be divided into two steps; a regional wind assessment, which evaluates the resources of a region as a first approach, and a site-specific assessment, which is a detailed evaluation of specific areas that exhibit high potential in the regional assessment [6].
The regional wind assessment is mostly based on numerical weather prediction (NWP) models [7-9]. Due to the high spread of wind power in the electricity system, the accuracy of wind prediction systems becomes increasingly important [10,11], have indicated that accurate wind forecasting is an essential process for modern electrical networks. In addition, a small error or little change in the accuracy of the wind speed forecast can reflect a high error in the wind power forecast [12,13], where the wind power is proportional to the cube of the wind velocity. Therefore, accurate wind forecasts save billions of dollars per year. In the absence or scarcity of wind speed measurements, alternative techniques and methods are required to assess and estimate wind resource availability, one of the most important studies in this application was performed by [14,15]. It should provide, at a minimum, an accurate average wind speed and power density prediction to better estimate the annual energy production [16]. The scaling techniques typically estimate the average wind speeds, while the power density can be estimated using the distribution of site wind speed [17]. Numerical Weather Prediction (NWP) datasets for wind speed are available in several resolutions and heights but must be scaled to provide more accurate near-surface wind speed [18].
Current NWP models resolve several local and regional circulation patterns, considering local topographic surface features to a certain extent. Thus, these models can provide accurate information for meteorological parameters, such as wind speed and direction, over large regions at high temporal and spatial resolution [19]. Predicting wind speed is a challenging task due to its inherent characteristics such as non-linearity and randomness. Modern NWP models have adopted sophisticated microphysics schemes that can depict the fine-scale atmospheric processes affecting heat, moisture, and momentum transfers. NWP models take into consideration the effects of the earth’s surface features and properties. These features have substantial effects on local weather characteristics, especially within the mixed boundary layer. The use of NWP data scaled by the boundary layer scaling (BLS) model could offer improved nearsurface wind speed and power density predictions compared to the use of the reference datasets [20]. Three dimensions weather simulations can provide detailed descriptions of atmospheric motions occurring over complex mountainous terrains [21]. Limited-area high-resolution NWP models can deliver good wind estimates in an approximately temporal range of 6 hours out to 6 days [22]. High-resolution nested models can describe fine-scale atmospheric phenomena, and their results have gradually become competitive with synoptic observations.
Non-hydrostatic weather forecasting models using nesting runs can supply good estimates of the wind conditions at local and simple synoptic scales when their results are compared to wind measurements [23]. Many numerical studies of wind resource assessment in different regions have compared the estimated wind velocity with the measured ones, and the comparisons showed a good agreement [24,25]. Increasing the resolution of numerical simulations along with the three-dimensionality representation of the topography can improve the model solution [26]. In recent years, many scientists have conducted a lot of research on the prediction of wind power. The Weather Research and Forecasting (WRF) model is one of the most widely used NWP models and is a reference for regional wind resource assessment [27,28]. The estimation of wind speed at 60 m (which is typically the height of the turbine hub) with the high horizontal resolution has been analysed by coupling of WRF mesoscale model and the Wind Atlas Analysis and Application Program (WASP) micro-scale model [29]. Also, the WRF model produces good results in wind speed predictions at 60 m in comparison to Final Analysis (FNL) wind speed data at 10 m using power law in Tanzania [30].
Several studies indicated that the configuration and scheme selection into the NWP model for wind simulation is regiondependent [31-35]. In a while, the initial and lateral boundary conditions, the model spin-up time [36,37], the parameterization of the influence of turbulence on wind velocity components [38] and planetary boundary layer (PBL) schemes [39] are the main dependant factors for the low-level wind’s accurate simulation at high resolutions in the NWP models. Therefore, a set of configurations in the NWP model must be determined for mapping the spatial wind resources [40,41] studied the effect of four modifications to the WRF model during eight simulation experiments. The modifications included Radio occultation data assimilation, different versions of the WRF model, utilizing bulk microphysics schemes that have been established in the period from 1983 to 2011, and completely coupling WRF to the ocean dynamic model. The accuracy of the simulation has been evaluated through a comparison between each simulation to the Global Forecasting System (GFS) model analysis [42], studied the evaluation of nonconvective wind forecasting methods in the 15th operational weather squadron area of responsibility.
The study assessed two different techniques for forecasting non-convective wind gusts at different five positions. Using a WRF ensemble data set, the results indicated that the Rapid Update Cycle’s wind gust algorithm (RUC) led to a better performance at three of the five locations when evaluated with more than the 35- knot threshold. The results of this study indicated that integration between two non-convective wind-gust prediction techniques into operational forecasts at the 15th operational weather squadron could give better accuracy with more evaluation and testing against other accepted techniques. The spatial and temporal high-resolution of wind velocity observations are insufficient in Egypt, Sudan, and South Sudan. Hence, they could overcome this problem using numerical weather prediction models. The windenergy resource evaluation needs long historical wind speed and direction observations. The observed wind data are not adequate to study the national wind resource over Egypt, Sudan, and South Sudan. This study proposed to use the WRF model, as one of the new-generation numerical weather prediction models that include advanced dynamics, physics, and numerical schemes and is suitable for a broad spectrum of applications across scales ranging from meters to thousands of kilometres. The basic reason for selecting the WRF model is its dynamic core, the physical framework, and the software framework. The major goal of this study is to evaluate regionally the performance and effectiveness of the WRF model in predicting wind over Egypt, Sudan, and South Sudan to confirm its capacity to provide accurate wind data in comparison to observations.
Egypt, Sudan, and South Sudan are located in the northeast of Africa, and they are bounded by latitudes from 3 to 32°N and longitudes from 21 to 39°E. The study area with plotted weather stations (ten in Egypt and nine in Sudan and South Sudan) is shown in Figure 1. The detailed geographical information for the selected stations is presented in Tables 1 & 2.
Table 2: Sudan and South Sudan stations, their IDs, latitudes, longitudes, elevations, and WMO numbers.
The Advanced Research Weather Research and Forecasting (WRF-ARW) model version 3.8.1 is used to perform threedimensional real-time high-resolution nested experiments to estimate the wind speed over the two countries. The six-hourly reanalysis datasets from (i) the National Centre for Environmental Prediction/National Centre of Atmospheric Research (NCEP/ NCAR) with 2.5° X 2.5° spatial resolution and 18 vertical pressure levels; and (ii) from the Global Forecast System Final Analysis (GFSFNL) with 1°x1° horizontal resolution and 27 vertical pressure levels are used as the initial and lateral boundary conditions to run WRF model. In addition, the observed wind speed data is collected from the selected 19 ground stations inside Egypt, Sudan, and South Sudan and used to evaluate the WRF model performance for wind prediction. During this study, the WRF model is run on three nest domains with horizontal resolutions of 30 km (Domain one, DM1), 10 km (Domain 2, DM2), and 3 km (Domain 3, DM3) using the two-way nesting option. The third nested domain (DM3) of 3 km grid spacing is centered on the study area (Egypt, Sudan, and South Sudan, Figure 2 and used as the main output to achieve the purposes of this research.
By applying some statistical analysis and procedures on the observed wind speed data at the selected stations in Egypt, Sudan, and South Sudan during the period 1981-2010, it is found that the days of 22 January 2010, 26 April 1999, 12 September 1989, and 7 November 2003 contain the maximum wind speed in winter, spring, summer, and autumn respectively. Accordingly, four WRF sensitivity experiments were carried out on these days to investigate the performance of the WRF model during the different seasons. Based on data availability, the WRF model is forced by the NCEP/NCAR reanalysis dataset. Two days while running the WRF simulations were recognized as a spin-up time to adequately develop mesoscale processes. The WRF model outputs were proceeding every hour with 27 pressure levels and the model top of 50 hPa. The selected WRF model physics schemes in this study are shown in Table 3. The purpose of the present work was to investigate the success and accuracy of the WRF model based on the selected physics schemes in predicting wind speed over the study area, comparable to the ground station measurements. However, for a deeper understanding of the results, it is beneficial to have some idea of the accuracy at which the model can simulate wind speed for different regions in Egypt, Sudan, and South Sudan. Thus, statistical analysis procedures were executed to compare the WRF model and observations at the selected 19 ground stations.
To investigate the goodness of fit measurements between the measured and predicted wind speed for the selected stations, the following statistical analysis methods were used.
Willmott has developed an index to measure the degree of difference between model predictions and observations; its value varies between 0 and 1 [43]. When the difference is 1, it represents a perfect match between the predictions and observations. While if it is 0, it indicates that there is no agreement at all. It is given by equation (1):
Where Xi, and Yi represents the observed, observed average, and simulated values respectively.
Coefficient of Determination (R2)
The coefficient of determination is an indicator that assesses how well a model predicts projected outputs. Also, it represents the degree of variation in the dataset. R2 value ranges between 0 and 1 because it is the square of the correlation between the modeled and measured variables. Where higher values indicate less error variance and that the model has a good fit and values greater than 0.5 are considered acceptable [44,45]. The R2 is given by equation (2):
Normalized Root Mean Square Error (NRMSE)
It is considered one of the very important statistical tools which give a good overall measure of model performance. The weighting of prediction-observation by its square tends to inflate Root Mean Square Error (RMSE), particularly when extreme values are present. Concerning a perfect model, the RMSE should approach zero [46]. RMSE is normalized by dividing its values by the average observed and multiplying by 100 [47-49], this formula is given by equation (3):
Where n represents the number of observed and predicted values used in the comparison.
Normalized Mean Bias Error (NMBE)
The Mean Bias Error (MBE) is considered to evaluate the model performance and measure the systematic error between the predicted and observed values [50]. Lower numbers are best and values less than 0 indicate under-prediction. It is also normalized by dividing its values by the average observed and multiplying by 100 (NMBE) [51]. It is given by equation (4):
Figure 3 shows a comparison between measured and predicted wind speed for the selected stations during spring (26 April 1999). One may notice that the WRF model reveals a good performance and is in harmony compared to the observed wind speed at all stations during spring. But the predicted wind speed over all stations is slightly greater than the observed one (overestimations). Figure 4 shows a comparison between measured and predicted wind speed for the selected 19 stations in Egypt, Sudan, and South Sudan during winter (22 January 2010). The predicted values of wind speed using the WRF model have the same symmetry and behavior as the observed one. In addition, the difference between the predicted and observed wind speed may be reached zero over most stations. Hence, the performance of the WRF model in predicting wind speed in the winter is better than in spring. The comparison between measured and predicted wind speed for the selected stations in Egypt, Sudan, and South Sudan during summer (12 September 1989) is shown in Figure 5. It was observed that the pattern of the predicted wind speed is similar in shape and harmony with the observed one over all stations.
Figure 3: Comparison between measured and predicted wind speed during spring for selected stations: (A): Egypt stations, (B): Sudan, and South Sudan.
Figure 4: Comparison between measured and predicted wind speed during winter for selected stations: (A): Egypt, (B): Sudan, and South Sudan.
Figure 5: Comparison between measured and predicted wind speed during summer for selected stations: (A): Egypt, (B): Sudan, and South Sudan.
Figure 6 shows a comparison between measured and predicted wind speed for (A) Egypt stations, (B) Sudan, and South Sudan during autumn (7 November 2003). The predicted values of wind speed using the WRF model have the same symmetry and behavior as the observed one. In addition, the differences between the predicted and observed values are very small over most stations. Hence, the performance of the WRF model in predicting wind speed in autumn is very good and mostly like summer and winter. Therefore, the highest performance of the WRF model in predicting wind speed could be arranged from highest to lowest in summer, autumn, and winter and slightly decrease in spring. Overall, it may conclude that; Egypt, Sudan, and South Sudan can depend on the WRF model to predict wind speed in the future with high confidence. In addition, Tables 4 & 5 show small differences between measured and predicted wind speed at the selected stations. It is noticed that the WRF model wind speed average was overestimated compared to the measured one at all stations with a maximum difference in the spring season reached at above 2 m/sec. Furthermore, the comparisons between predicted and measured wind speed at each season and station have different behavior in prediction than others, but overall, the model results and prediction errors are relatively acceptable with a maximum systematic difference during the spring season.
Figure 6: Comparison between measured and predicted wind speed during Autumn for selected stations: (A): Egypt, (B): Sudan, and South Sudan.
From a statistical point of view, the results in a Table 6 indicated that the predicted wind speed values were close to the measured ones. The goodness of fit test revealed a significant convergence between the measured and predicted values. Where R2 and WI values are closer to 1 for all seasons except summer in Egypt, Sudan, and South Sudan. While the NRMSE values ranged from 9.715% during autumn to 16.880% during spring in Egypt and from 6.470% during winter to 17.040% during spring in Sudan. Furthermore, NMBE values simulated the same behavior of NRMSE with maximum bias during spring in Egypt, Sudan, and South Sudan with 12.755% and 15.181% respectively. These results are confirmed in Tables 4 & 5. The results in Table 6 showed that the best goodness occurred during autumn in Egypt and winter in Sudan and South Sudan. While the lowest correlation between observed and predicted wind speed was during summer in Egypt, Sudan, and South Sudan. It was noticed that there are no significant differences between the observed wind speed and the WRF forecasts during all seasons, which confirms that the WRF model success and has a good ability and high performance to simulate and predict wind speed during the different seasons over the three countries.
The WRF wind speed prediction was in harmony comparable to the observation over the study area (Egypt, Sudan, and South Sudan) with high performance during all seasons except spring. The highest values of WI and R2 were close to 1 and the smallest ones of NRMSE and NMBE were close to zero, thus there was a strong correlation between predicted and measured wind speed. In addition, there were no significant differences between the actual and forecasted wind speed for the selected days. Therefore, the WRF model is competent to predict wind speed over Egypt, Sudan, and South Sudan. The highest performance of the WRF model was in autumn in Egypt and winter in Sudan, South Sudan, where the NRMSE values were small. While its lowest one was found in summer compared to observation due to its maximum difference and highest NRMES. Overall results proved the capability of the WRF model to predict wind speed over Egypt, Sudan, and South Sudan compared to measurements. In general, it may conclude that; Egypt, Sudan, and South Sudan can depend on the WRF model to predict wind speed in the past, present, and future with relatively high confidence and accuracy.
Bio chemistry
University of Texas Medical Branch, USADepartment of Criminal Justice
Liberty University, USADepartment of Psychiatry
University of Kentucky, USADepartment of Medicine
Gally International Biomedical Research & Consulting LLC, USADepartment of Urbanisation and Agricultural
Montreal university, USAOral & Maxillofacial Pathology
New York University, USAGastroenterology and Hepatology
University of Alabama, UKDepartment of Medicine
Universities of Bradford, UKOncology
Circulogene Theranostics, EnglandRadiation Chemistry
National University of Mexico, USAAnalytical Chemistry
Wentworth Institute of Technology, USAMinimally Invasive Surgery
Mercer University school of Medicine, USAPediatric Dentistry
University of Athens , GreeceThe annual scholar awards from Lupine Publishers honor a selected number Read More...